In the rapidly evolving digital world of artificial intelligence (AI), Large Language Models (LLMs) have become a focal point of discussion due to their remarkable capabilities in generating text, translating languages, and answering questions in a highly informative manner. But a critical question lingers: Can these sophisticated models understand and process visual concepts without direct exposure to images?
A groundbreaking study conducted by researchers at MIT’s Computer Science and Artificial Intelligence Laboratory (CSAIL) provides an affirmative answer to this question. The research reveals that LLMs, although primarily trained on text, possess a substantial amount of hidden visual knowledge. This discovery opens up new avenues for utilizing LLMs in generating images, recognizing objects in photos, and even training computer vision systems.
Unveiling the Hidden Visual Knowledge of LLMs
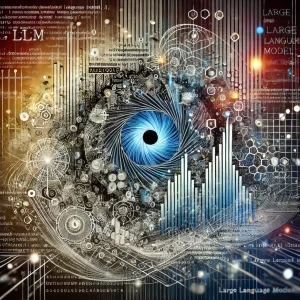
The primary objective of the CSAIL study was to explore whether LLMs, which have not been explicitly trained on visual data, could comprehend and generate visual concepts. To investigate this, the researchers employed a novel approach: they used LLMs to generate images through code, assessing their ability to understand and render visual elements such as shapes, objects, and scenes.
LLMs and Image Generation
The results of the study were nothing short of extraordinary. The LLMs were able to produce intricate and creative images, showcasing a deep understanding of visual concepts despite never having been directly trained on actual images. The researchers tested the models by asking them to draw various objects such as chairs, cars, and lamps. Not only did the LLMs successfully render these objects, but they also incorporated detailed visual effects like shadows and reflections, which are typically associated with direct visual experience.
Abstract Concepts and Visual Representation
One of the most fascinating aspects of the study was the ability of LLMs to grasp abstract concepts and translate them into visual form. For instance, when prompted to depict ideas like “feeling happy” or “the sound of music,” the LLMs produced images that encapsulated these notions through the use of symbols and metaphors. This ability to visualize abstract concepts suggests that LLMs can understand and manipulate complex visual ideas, potentially transforming how we approach AI-driven creativity.
Leveraging Visual Knowledge for Computer Vision
The implications of LLMs‘ hidden visual knowledge extend far beyond mere image generation. In a significant advancement, the CSAIL researchers used the visual understanding embedded within LLMs to train a computer vision system. They did this by first generating a dataset of images using the LLMs, which included various objects and scenes, and then using this dataset to train a computer vision model.
Enhanced Object Recognition
The performance of the computer vision system trained with LLM-generated images was compared to systems trained with real-world images. Remarkably, the system trained with the LLM-generated images outperformed its counterparts. This finding indicates that the visual knowledge harbored by LLMs can be effectively harnessed to improve the accuracy and efficiency of computer vision systems. This could lead to significant advancements in fields where computer vision is critical, such as autonomous vehicles, medical imaging, and facial recognition.
The Broader Implications of CSAIL’s Findings
The research from CSAIL represents a pivotal moment in our understanding of LLMs and their capabilities. It demonstrates that these models are not limited to text-based tasks but can also engage with and understand visual concepts, making them more versatile than previously thought.
Potential Applications
The potential applications of this discovery are vast and varied. For instance, LLMs could be used to create more intuitive and visually rich user interfaces, enhancing user experiences across a range of platforms. Additionally, the ability of LLMs to generate detailed images from abstract concepts could be leveraged in the creation of new forms of digital art, pushing the boundaries of AI-driven creativity. Moreover, the improvement in computer vision systems trained with LLM-generated images could lead to more accurate and reliable AI applications in industries such as healthcare, security, and retail.
Artistic Innovation
One particularly exciting possibility is the use of LLMs in the arts. By generating images from textual descriptions, LLMs could serve as collaborators in artistic creation, offering new perspectives and ideas that might not occur to human artists. This could lead to the emergence of a new genre of AI-assisted art, where human creativity is augmented by the unique visual interpretations of LLMs.
Limitations and Future Challenges
Despite the promising results of the CSAIL study, there are still several challenges and limitations that need to be addressed. For one, while LLMs have shown an impressive ability to generate and understand visual concepts, they are not infallible. There are instances where LLMs struggle to accurately recognize and represent visual elements, leading to images that may be technically correct but lack the nuance and depth of real-world visuals.
Understanding the Mechanisms Behind Visual Knowledge
Another significant challenge lies in understanding how LLMs acquire their visual knowledge. Since these models are trained on vast amounts of text data, the process by which they internalize and process visual concepts is not yet fully understood. Further research is necessary to unravel the underlying mechanisms that enable LLMs to perform visual tasks and to refine these models for even greater accuracy and efficiency.
Ethical Considerations
As with all advancements in AI, there are ethical considerations that must be taken into account. The ability of LLMs to generate realistic images raises questions about the potential for misuse, such as the creation of deepfakes or the generation of misleading visual content. It is essential that researchers and policymakers work together to establish guidelines and safeguards to prevent the misuse of this technology while maximizing its positive impact.
Conclusion
The research conducted by MIT’s CSAIL marks a significant step forward in our understanding of the capabilities of Large Language Models. It reveals that LLMs possess hidden visual knowledge that can be utilized not only for generating images but also for training computer vision systems and understanding abstract concepts. These findings have far-reaching implications for the future of AI, opening up new possibilities for innovation across various industries.
As we continue to explore the potential of LLMs, it is crucial to address the challenges and ethical considerations that accompany these advancements. With further research and responsible development, LLMs could revolutionize the way we interact with and understand both text and visuals, leading to a new era of AI-driven creativity and intelligence.